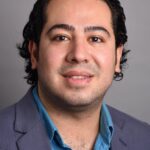
Tuesday, 09/28/2021
2:00 PM
Zoom Link: https://ccny.zoom.us/j/89080559357
Professor Safa Jamali
Northeastern University, Mechanical and Industrial Engineering
“Rheology-Informed Neural Networks (RhINNs) for Complex Fluid Modelling”
ABSTRACT
Reliable and accurate prediction of complex fluids’ response under flow is of great interest across many disciplines, from biological systems to virtually all soft materials. The challenge is to solve non-trivial time and rate dependent constitutive equations to describe these structured fluids under various flow protocols. On the other hand, advances in data-driven approaches to material design and discovery promise a leap in accelerated design cycles for new materials. I will present Rheology-Informed Neural Networks (RhINNs) as a general platform for prediction of rheological behavior in complex fluids. This includes a neural network architecture capable of solving Ordinary Differential Equations (ODEs) adopted for complex fluids, in forward and reverse problems, as well as a multi-fidelity approach in which scarcity of experimental data is compensated by readily-available model predictions to train the machine learning platform. The proposed RhINNs are employed to solve the constitutive models with multiple ODEs by benefiting from Automatic Differentiation in neural networks. We present direct and inverse solutions of a Thixotropic Elasto-Visco-Plastic (TEVP) constitutive equation for a series of different flow protocols by employing our RhINNs methodology. I will show that the RhINNs framework is capable of learning complex model parameters by training on a series of limited experimental data. We show that the model can be extended to various models by including different systems of ODEs, solved for arbitrary geometries, and recover complex kymographs of kinematic heterogeneities and transient shear banding of thixotropic fluids. Finally, several Rheology-Informed Neural Networks (RhINNs) architectures as alternative platforms for constitutive meta-modeling of complex fluids and the outlook will be discussed.
BRIEF ACADEMIC/EMPLOYMENT HISTORY:
I received my bachelor’s degree from Amirkabir University of Technology in Iran, followed by a master’s degree from Catholic University of Louvain, in Belgium. After that I moved to Cleveland, OH, for my PhD work in the department of Macromolecular Science of Case Western Reserve University. After receiving my PhD in 2015, I went to MIT for postdoctoral training in departments of chemical and mechanical engineering. I joined the department of mechanical and industrial engineering and Northeastern University in 2017, and have been there since.
MOST RECENT RESEARCH INTERESTS:
My research interests span from physics and rheology of particulate systems, to hemorheology and hemodynamics, and to developing science-based data-driven techniques for complex material systems.